Центар за изучавање комплексних система организује SCL семинар у четвртак, 8. марта 2018. у 14 часова у читаоници библиотеле „Др Драган Поповић“. На семинару ће говорити Владимир Глигоријевић са Flatiron института у Њујорку, САД.
Тема семинара је: „Deep Multi-network Embedding for Protein Function Predictiction“.
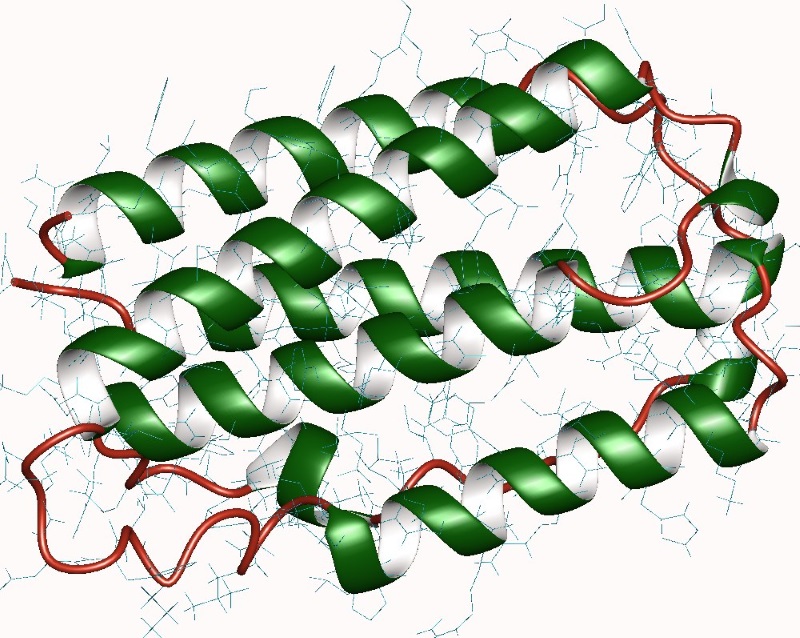
АПСТРАКТ:
The prevalence of high-throughput experimental methods has resulted in an abundance of large-scale molecular and functional interaction networks. The connectivity of these networks provides a rich source of information for inferring functional annotations for genes and proteins. An important challenge has been to develop methods for combining these heterogeneous networks to extract useful protein feature representations for function prediction. Most of the existing approaches for network integration use shallow models that cannot capture complex and highly-nonlinear network structures. We introducedeepNF, our novel deep-learning based network integration method for protein function prediction. deepNF consists of two steps: 1) creating a low-dimensional dense vector representation of proteins (i.e., embedding) using Multimodal Deep Autoencoders and 2) training a classifier on the resulting representation to predict protein functions.
We apply deepNF on 6 different networks obtained from the STRING db to construct a compact low-dimensional representation containing high-level protein features. We will present an extensive performance analysis comparing our method with the state-of-the-art network integration methods for protein function prediction. In addition to cross-validation, the analysis also includes a temporal holdout validation evaluation similar to the measures in Critical Assessment of Functional Annotation (CAFA). Our results show that our method outperforms previous methods for both human and yeast STRING networks. Our method offers a great advantage of being able to capture non-linear information conveyed by large-scale biological networks, leading to improved network representations. Features learned by our method lead to substantial improvements in protein function prediction accuracy, which could enable novel protein function discoveries.