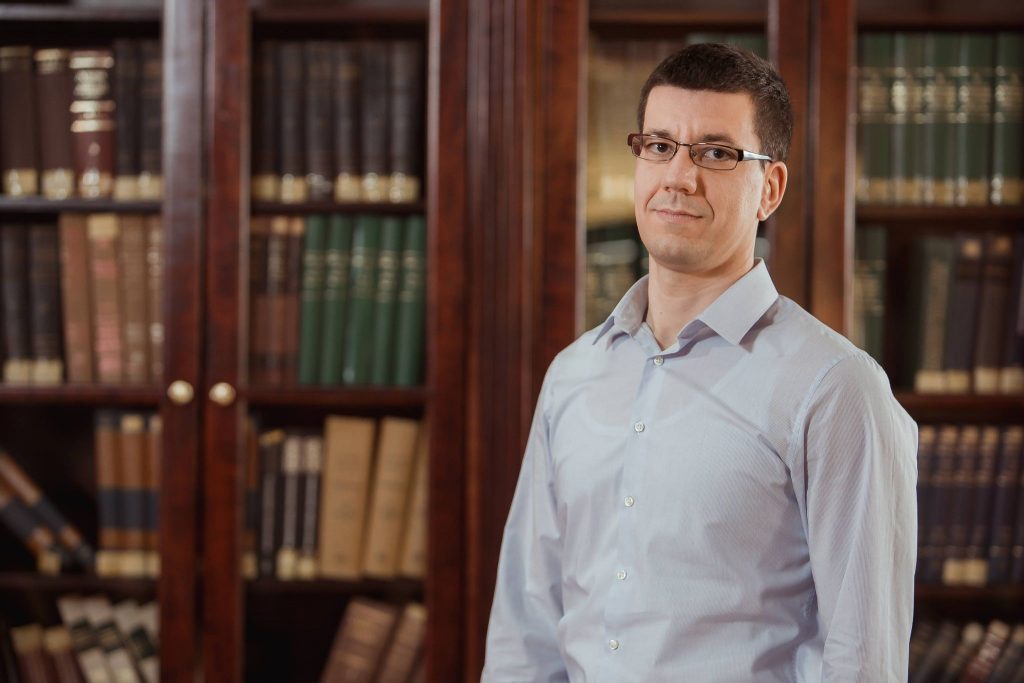
A team of researchers from CERN, Google and the Institute of Physics, Belgrade published a paper in June 2021, in Nature Machine Intelligence Journal presenting a new technique of machine learning, which could improve the detection of particle collision at the Large Hadron Collider. One of the authors of the paper is Dr Vladimir Lončar of the Institute of Physics, Belgrade, who has worked at CERN for the last several years as an information technology specialist researching and developing advanced systems of machine learning.
Dr Lončar is a member of the CERN team which specifically deals with the application of machine learning on the systems for triggering the Large Hadron Collider. ’These systems consist of unique hardware called field-programmable gate arrays (FPGA) tasked with quickly choosing whether some event is for further processing or discarding, based on the detector data’, explains Dr Lončar, adding that more than 99% of events are discarded.
As stated on the official CERN website, machine learning has found multiple applications in particle physics, and the new technique, which was developed by the authors of the paper, will make it possible to apply deep neural networks on systems for early triggering in the process of selecting events for further analysis. The main challenge during the development of the ’Trigger’ system is the rate of proton-proton collisions, which is so great (up to 1 billion collisions per second) that it is necessary to recognize a potentially interesting event, and choose whether to save it or discard it in mere microseconds. In Dr Lončar’s words, the new method will enable us to replace the existing manually adjusted algorithms with optimized neural networks, thus improving the decision-making system. ’It will reduce the number of events of interest that we miss, which allow us new research and a better understanding of what the Universe is made of’, states Dr Lončar.
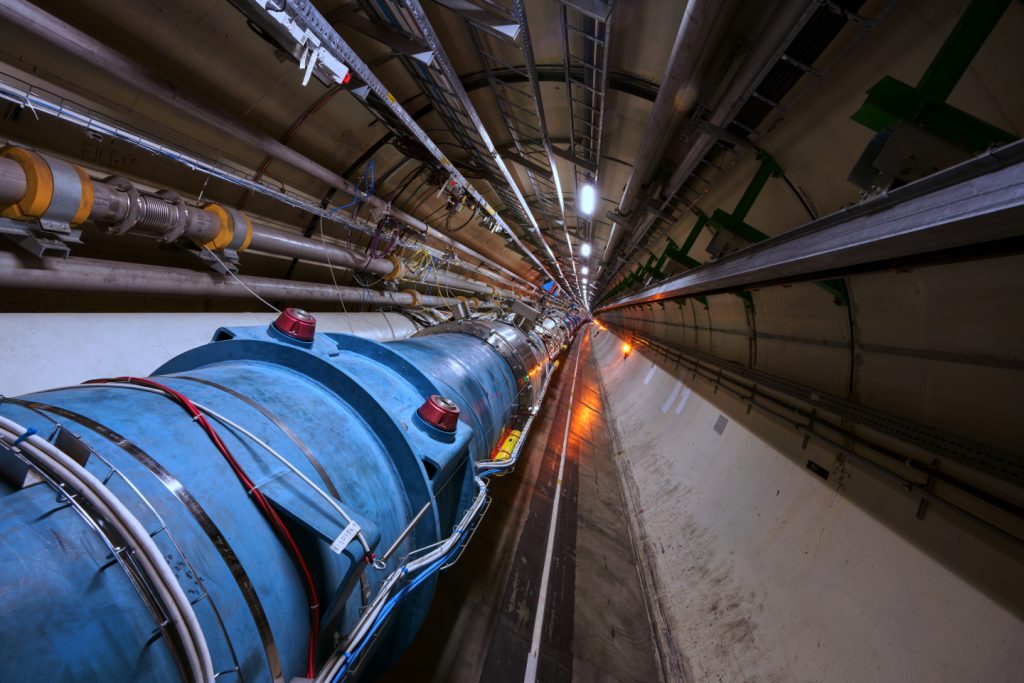
In the abstract of the paper, the authors state that in the search for better solutions, the research in the fields of machine and deep learning leans towards more complex models, but the model size and its computing complexity should be somehow limited if we are to apply these methods to the systems with limited computational capacity. One technique researchers studied to limit model size is quantization, i.e. using fewer bits to represent the data in the model. However, careless reduction of the number of bits usually results in a decline in the model performance, i.e. errors in the model’s choices. Therefore, the new method simplifies the development of quantized models with minimized loss in performance. ’The goal is to create the best quantization system for a given model through the automatized process, thus facilitating the work of the person developing it’, clarifies Dr Lončar.
The researchers from CERN want to develop efficient models which would most effectively use hardware uniqueness on which they are deployed, specifically on FPGA devices used in the trigger system. For these systems to make a decision in a limited period of time, it is necessary to reduce the amount of the model’s computational operations. ’We have developed a platform which allows efficient deploying of models on FPGA hardware, but since the models are developed on conventional computers, in the process of their translation to FPGA, they lose accuracy or require too many computational operations’, says Dr Lončar, adding that it was necessary to come up with a tool that would allow model development optimized in advance for this hardware. This was precisely the reason for teaming up with the Google team.
The method developed by the team of researchers, whose member is Dr Vladimir Lončar, could find its application beyond the solution of a particular problem of an experiment at CERN. It could find its use in experiments in high-energy physics, as well as in industry, therefore the researchers have already discussed the application of this technology with partners from the vehicle industry. Dr Lončar states ’We can apply the developed techniques and tools in all spheres where it is necessary to process data as soon as possible on hardware with limited computer capacities, such as cars or mobile phones. We can make these devices more autonomous or smarter while eliminating the need for a constant connection to the Internet.